Fintech: Understanding AI Use in Financial Services
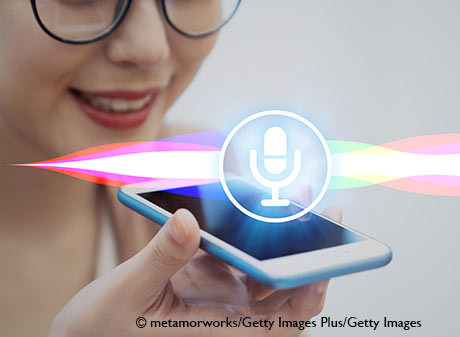
This post is part of a series titled “Supervising Our Nation’s Financial Institutions.” The post was written by Julie Stackhouse, then-executive vice president and officer-in-charge of supervision at the St. Louis Fed. She is now retired.
In February, I provided an overview of the technological revolution occurring in the banking industry. Financial technology, or fintech, is being adopted by financial institutions of all sizes as well as nonbank providers of financial services. Over the next few months, I’ll examine how a number of fintech applications are being used in banking. This post covers artificial intelligence and two of its branches:
- Machine learning (ML)
- Natural language processing (NLP)
Have you asked Siri a question? Have you driven a Tesla? If so, you’ve been the beneficiary of artificial intelligence, or AI!
The Financial Stability Board defines AI as “the theory and development of computer systems able to perform tasks that traditionally have required human intelligence.” “Artificial intelligence and machine learning in financial services,” Financial Stability Board, Nov. 1, 2017. It sounds complicated, but some forms of AI—like Google’s search engine—have been used for decades. Newer types of AI—such as ML and NLP—have emerged more recently as aggregation of massive amounts of data (often called “big data”) has become easier and the use of faster, more powerful computing has spread.
How do these newer technologies work? ML perfects rules-based computer programs (called algorithms) automatically with limited or no human intervention. NLP helps computers understand, interpret and manipulate human language. The use of NLP—along with advanced machine learning called “deep learning”—allows a voice assistant such as Siri to recognize and respond to spoken language.
Banking Industry Use of AI
The banking industry is increasingly taking advantage of AI, especially ML. Examples include:
Fraud Detection
ML algorithms can sort through a customer's history of transactions-amounts, location, time and merchant or vendor-to see whether a current transaction is an outlier and flag it for investigation.
Credit Extensions
ML can sift out borrower characteristics from nontraditional sources such as utility bills, cellphone usage and social media footprints. These data supplement the usual underwriting criteria of credit scores and debt payments.
Bank Secrecy Act Compliance
ML algorithms can help banks with the expensive, labor-intensive and duplicative work required to know their customers. The technology evaluates images to match documents and then flags those that might merit extra scrutiny. The use of fintech in regulatory compliance has been dubbed “regtech.”
Personalized Service
ML algorithms can predict likely product selection based on a customer’s financial data, habits and spending style. Voice-enabled “chatbots”—computer programs designed to simulate human conversation—are frequently employed for this task, powered by ML programs that use NLP.
Risks of AI in Banking
While AI tools provide potentially significant value for banks, there are risks involved. In lending, for example, bankers must be careful that algorithms do not intentionally or unintentionally discriminate on a prohibited basis. The use of advanced technology can also increase a bank’s cyber footprint and, accordingly, its cyber risk posture.
Further, banks that build AI models have the responsibility to validate those models, and those who procure models through vendors must ensure they understand the risk acceptance decisions of the vendors. And finally, AI-based lending models have yet to be tested in an economic downturn.
Whenever new technology is employed, there are certain to be some challenges along the way. But given its great promise, technology such as AI will increasingly be deployed by banks and nonbanks alike.
Notes and References
1 “Artificial intelligence and machine learning in financial services,” Financial Stability Board, Nov. 1, 2017.
Additional Resources
- On the Economy: The Basics of Fintech
- On the Economy: A Glimpse into Banking’s Future by Looking at the Past
- On the Economy: Fintech Interest in Industrial Loan Company Charters: Spurring the Growth of a New Shadow Banking System?
Citation
Julie L Stackhouse, ldquoFintech: Understanding AI Use in Financial Services,rdquo St. Louis Fed On the Economy, April 28, 2019.
This blog offers commentary, analysis and data from our economists and experts. Views expressed are not necessarily those of the St. Louis Fed or Federal Reserve System.
Email Us
All other blog-related questions