The Rapid Adoption of Generative AI
Generative artificial intelligence (AI) has rapidly emerged as a potentially transformative workplace technology. The large language model (LLM) ChatGPT debuted in November 2022, and by March 2024 the most common generative AI tools were being accessed by hundreds of millions of users each month. While several studies have found that generative AI can result in sizeable productivity gains for workers, firms’ adoption of the technology in production has been reported to be fairly low.See, for example, Aakash Kalyani and Marie Hogan’s April 2024 On the Economy blog post, “AI and Productivity Growth: Evidence from Historical Developments in Other Technologies.” The overall effect of generative AI on the economic landscape hinges on how many people adopt the new technology and how intensively they use it.
In this blog post, we present results from the first nationally representative U.S. survey of generative AI adoption at work and at home. Our data come from the Real-Time Population Survey (RPS), a nationwide survey that asks the same core questions and follows the same timing and structure as the Current Population Survey (CPS), the monthly labor force survey conducted by the U.S. Census Bureau for the Bureau of Labor Statistics. We have used the RPS previously to study the rise in work from home since the onset of the COVID-19 pandemic.See, for example, the June 2024 On the Economy blog post “The Impact of Work from Home on Interstate Migration in the U.S.”
How Prevalent Is Generative AI Adoption?
As shown in the figure below, the RPS revealed that in August 2024 almost 40% of the U.S. population ages 18 to 64 used generative AI to some degree, and almost 1 in 3 respondents said they used it daily or at least once but not every day during the week prior to the survey. Usage at home was somewhat more prevalent than at work (32.6% versus 28.1%, respectively), but daily usage was less frequent at home than at work (6.4% versus 10.6%, respectively).
Use of Generative AI at Work and at Home, August 2024
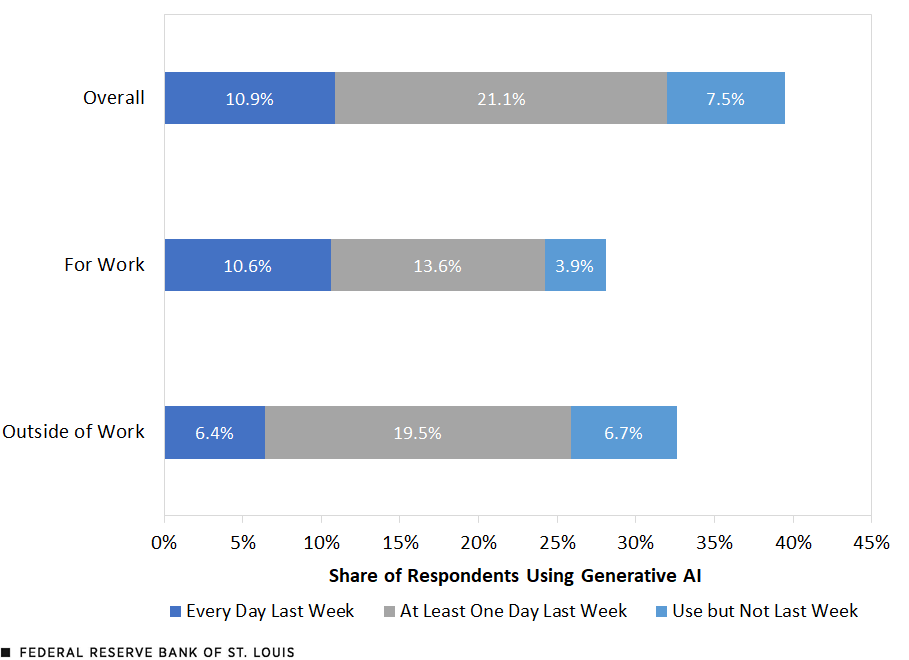
SOURCES: Real-Time Population Survey and authors’ calculations.
NOTES: The figure shows the share of RPS respondents who used generative AI for work, outside of work and overall (either for work or outside of work). Intensity of use is broken down into every day in the week before the survey, at least one day in the week before the survey but not every day, and not used in the week before the survey. Data are from the August 2024 wave of the RPS and for respondents ages 18 to 64. The “for work” sample includes only employed individuals (N=3216); the other samples include all respondents (N=4682).
In our working paper on this topic, we show that generative AI use is more common among individuals who are male, younger and more educated and who work in computer, math and management occupations. However, perhaps the most important finding is that generative AI use is widespread across gender, age, education, industries and occupations.
How Does Adoption of Generative AI Compare with That of Other Technologies?
The next figure compares the speed of adoption of generative AI with that of two other transformative technologies: personal computers (PCs) and the internet. We plot the adoption rate by year since the first mass market version of each technology. The first mass market computer was the IBM PC, which was released in August 1981 and sold more than a million units. We date mass market availability of the internet to April 1995, when the National Science Foundation (NSF) decommissioned NSFNET and allowed the internet to carry commercial traffic. The first generative AI model to eventually sell at least one million subscriptions (ChatGPT) was released in November 2022, roughly two years before the date of our survey data.
Adoption Rate of Generative AI at Work and Home versus the Rate for Other Technologies*
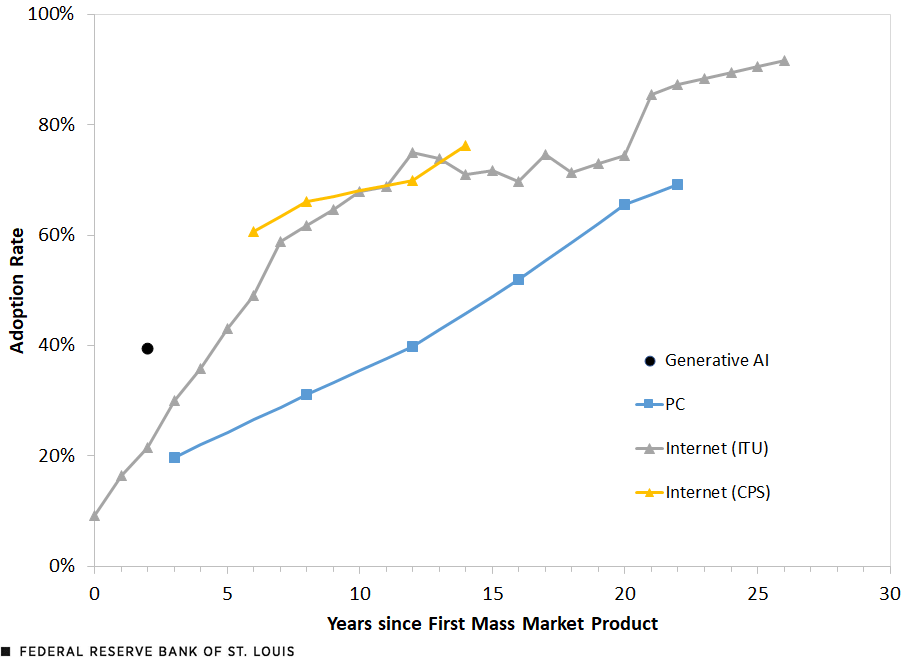
SOURCES: Real-Time Population Survey, Current Population Survey, International Telecommunication Union and authors’ calculations.
NOTES: The figure shows usage rates at work for three technologies: generative AI, PCs and the internet. The horizontal axis represents the number of years since the introduction of the first mass market product for each technology. AI usage data are from the August 2024 wave of the RPS. PC usage data are from the 1984-2003 Computer and Internet Use Supplement of the CPS. We plot two estimates of internet use: one from the 2001-09 Computer and Internet Use Supplement of the CPS and one with 1995-2021 data from the International Telecommunication Union (ITU). The samples from the RPS and CPS include all individuals ages 18 to 64. The RPS sample size is 4,682. The sample from the ITU includes individuals of all ages. The figure’s data are available for download.
The black dot in the figure above repeats the 39.4% adoption rate for generative AI reported in the first figure. Generative AI has been adopted at a faster pace than PCs or the internet. Adoption of the PC three years after its mass introduction was only at 20%, about the same value as for adoption of the internet after two years.
For What Tasks Do People Use Generative AI?
The RPS also asked respondents about how they used generative AI. Respondents who indicated that they had used generative AI in the last week were presented with the list of 10 tasks (plus an “other” category) shown in the following figure. They were then asked to select any of the tasks for which they used generative AI at work during that period. (In our working paper, we show similar results for generative AI usage at home.)
Types of Tasks for Which U.S. Workers Are Using Generative AI, August 2024
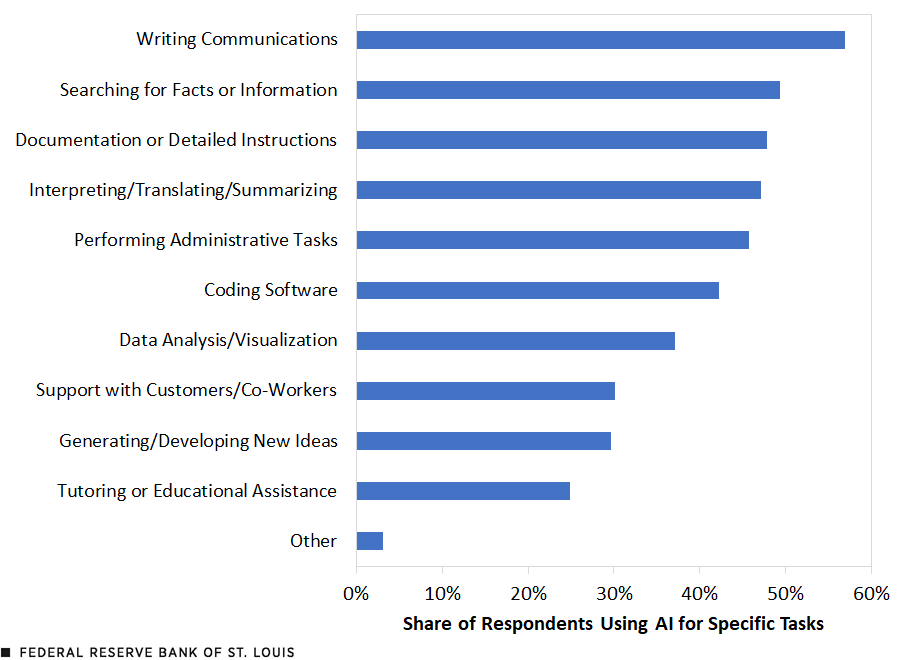
SOURCES: Real-Time Population Survey and authors’ calculations.
NOTES: The figure shows the share of AI users employing it for specific tasks at work. Data are from the August 2024 wave of the RPS and for respondents ages 18 to 64. The sample includes only employed individuals (N=3216). The figure’s data are available for download.
In a nutshell, respondents used generative AI for a wide range of tasks, with usage rates at or exceeding 25% for all 10 defined tasks. The survey also asks respondents to rank the tasks for which they used generative AI in order of how helpful the technology was in completing the task. Remarkably, the rankings were fairly evenly distributed overall, with eight of the 10 defined tasks being ranked among their top two selections by at least 10% of respondents.
How Much Could Generative AI Increase Labor Productivity?
Finally, we examined how intensely respondents employed generative AI on days that they reported using it. This allowed us to estimate a lower and upper bound for the share of total work hours that involve generative AI for the U.S. economy as a whole. We estimated that between 0.5% and 3.5% of all work hours in the U.S. are currently assisted by generative AI. Combining these estimates with a median increase of 25% in task productivity from the adoption of generative AI, an increase consistent with that observed in several studies,See, for example, the 2023 study “Experimental Evidence on the Productivity Effects of Generative Artificial Intelligence” by Shakked Noy and Whitney Zhang; the 2023 study “Generative AI at Work” by Erik Brynjolfsson, Danielle Li and Lindsey R. Raymond; the 2023 study “Navigating the Jagged Technological Frontier: Field Experimental Evidence of the Effects of AI on Knowledge Worker Productivity and Quality” by Fabrizio Dell’Acqua et al.; the 2024 study “The Effects of Generative AI on High-Skilled Work: Evidence from Three Field Experiments with Software Developers” by Zheyuan (Kevin) Cui, Mert Demirer, Sonia Jaffe, Leon Musolff, Sida Peng and Tobias Salz; and the 2023 study “The Impact of AI on Developer Productivity: Evidence from GitHub Copilot” by Sida Peng, Eirini Kalliamvakou, Peter Cihon and Mert Demirer. we estimated that generative AI could plausibly grow labor productivity by between 0.1% and 0.9% at current levels of usage.
Is Generative AI a General-Purpose Technology?
Generative AI has rapidly emerged as an important new technology, yet its impact on the U.S. economy depends critically on the speed and intensity of its adoption. We show that two years after the mass introduction of generative AI, its adoption rate already exceeds that of the PC and internet at comparable points in time. The wide variety of tasks for which people use generative AI, combined with the widespread adoption of generative AI across many demographics and occupations, suggest that it is a general-purpose technology.
*Editor’s Note: The second figure title was updated Oct. 10, 2024, to include “and home.”
Notes
- See, for example, Aakash Kalyani and Marie Hogan’s April 2024 On the Economy blog post, “AI and Productivity Growth: Evidence from Historical Developments in Other Technologies.”
- See, for example, the June 2024 On the Economy blog post “The Impact of Work from Home on Interstate Migration in the U.S.”
- See, for example, the 2023 study “Experimental Evidence on the Productivity Effects of Generative Artificial Intelligence” by Shakked Noy and Whitney Zhang; the 2023 study “Generative AI at Work” by Erik Brynjolfsson, Danielle Li and Lindsey R. Raymond; the 2023 study “Navigating the Jagged Technological Frontier: Field Experimental Evidence of the Effects of AI on Knowledge Worker Productivity and Quality” by Fabrizio Dell’Acqua et al.; the 2024 study “The Effects of Generative AI on High-Skilled Work: Evidence from Three Field Experiments with Software Developers” by Zheyuan (Kevin) Cui, Mert Demirer, Sonia Jaffe, Leon Musolff, Sida Peng and Tobias Salz; and the 2023 study “The Impact of AI on Developer Productivity: Evidence from GitHub Copilot” by Sida Peng, Eirini Kalliamvakou, Peter Cihon and Mert Demirer.
Citation
Alexander Bick, Adam Blandin and David Deming, ldquoThe Rapid Adoption of Generative AI,rdquo St. Louis Fed On the Economy, Sept. 23, 2024.
This blog offers commentary, analysis and data from our economists and experts. Views expressed are not necessarily those of the St. Louis Fed or Federal Reserve System.
Email Us
All other blog-related questions